Demystifying LLMOp’s: An Introduction to LLM Inference Serving (Part1)
LLMOps Series: Inference Serving
Series Links
Demystifying LLMOp’s: An Introduction to LLM Inference Serving (Part1) 👈
Hi everyone, this series will be focusing on a few key recipes that are helpful and required in setting up an enterprise scale large language models (LLMs) inference serving. The post is not about managed cloud based LLM hosting services (like AWS Bedrock or Databricks serving) but the discussed concepts are core for developing any such services. In this multiple post series, we will explore concepts like LLM models structures, inference libs, serving frameworks, API endpoints, multi-tenant architectures, token generation, input guard rails and many more other such concepts. So, without further delay, let’s begin with part 1 of the series.
Challenges in Creating Robust ML/AI Systems
The initial question we must ponder is why creating a scalable machine learning (ML) system poses a significant challenge?? 'Hidden Technical Debt in Machine Learning Systems' a seminal paper from Google, delves into the complexities of building robust ML systems. While machine learning models may appear powerful, they often come with hidden technical debt – issues that accumulate over time and hinder system maintainability. Sculley et al. expalin how ML models constitute only a small fraction of a much larger, complex system (as depicted in Figure 1). Issues such as data dependencies, evolving inputs, and poorly structured code can lead to system failures and substantial maintenance expenses. Unlike regular software, ML systems degrade over time due to data drift and unpredictable interactions. The paper recommends that quick fixes create long-term problems and suggests best practices like modular design, testing, and monitoring to keep ML systems reliable.
Although the fundamentals of ML system design remain the same, they have added additional complexities with the inclusion of large language models. LLMs are not only complex in structure but require massive compute, along with new functionalities like distributed deployment across nodes (GPU/CPU), prompt templates, careful training on huge infrastructure, guardrails, vector databases, semantic matching, and many more. Let's dig deeper into what it requires to host compute-heavy models like LLMs.
AIOps vs MLOps Vs LLMOps
With the advent of AI, and especially powerful models like LLMs, its reshaping system engineering. Terms like LLMOps, AIOps, and MLOps are becoming increasingly common, though they can often be a source of confusion. While these concepts overlap, they each address distinct aspects of managing AI systems. I am not going into too much detail here but hare are my two cents-
‘AIOps is the broadest application of AI to IT, MLOps is the application of operational principles to Machine learning models, and LLMOps is the application of operational principles to Large Language Models (Fig. 2).’
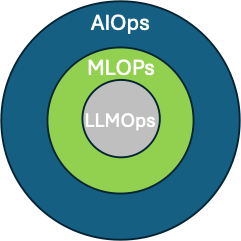
MLOps and AIOps: MLOps is a component of AIOps. For example, machine learning models deployed through MLOps can be used to perform anomaly detection or predictive maintenance in AIOps.
LLMOps and MLOps: LLMOps is a specialized form of MLOps. LLMs are machine learning models, so the general principles of MLOps apply here. However, LLMs have unique characteristics that require specialized tools and techniques.
Fig 3, explain the key differences-
I think we now have a good (or I would say basic 😊 ) understanding about initial broad terminologies. We can now go bit more in details about the LLM’s initialization.
LLM Inference and Serving
As previously discussed regarding all the Ops, Chip Huyen (author AI Engineering book) has summarized AI systems into a simplified three-layer strucutre known as the AI Engineering Stack- Application, Model and Infrastructure layer (Fig. 4). This post series will specifically focus on the Infrastructure stack and its model serving functionality. With the increasing prevalence of large language models such as GPT, LLaMA, and Mistral, a thorough understanding of their deployment nuances is essential. Two fundamental concepts in this area are model inference and model serving. Although these terms are often used interchangeably, they represent distinct components within the machine learning pipeline.
LLM Inference
Model inference is the process of using a trained machine learning model to generate predictions based on new input data. For LLMs, this means taking a user’s prompt (i.e. query) and producing a relevant text output. Inference is where the model applies its learned knowledge to answer queries, summarize documents, translate languages, or generate creative content.
LLM Model Download and File Structure
The first question is: where can we get all these LLM models? The short answer is beyond individual LLM providers, Hugging Face serves as a one stop shop. It is one of the premier platforms for downloading large language models (LLMs), offering access to state-of-the-art, open-source AI models, especially in natural language processing (NLP). It hosts thousands of pre-trained models like Mistral, Deepseek, along with specialized domain-specific variants such as FinBERT (for finance) domain. You can download models-
Directly clone repositories using Git lfs
Leverage the Python-based Hugging Face Hub library
Load models via integrated libraries like Transformers
Now let's see how a downloaded LLM model file looks like. Fig. 5 shows the directory structure of an "gemma-2-2b-it" which is a 2-billion-parameter version of the Gemma model from Google. Here’s what the different files represents:
In a nutshell-
Weights- (.safetensors) store the trained parameters.
Config files- (.json) define model architecture, tokenizer, and generation settings.
Tokenizer files convert text to tokens and back.
Metadata (README.md) provides usage details.
Fig. 6 depicts the original model size (weights+ biases). You can see the bigger model like Mistral 7B has weights around ~ 15GB, while smaller models (Gemma -2- 2B) size is around ~5GB. So, one can select different set of models depending on the compute capacity and use-cases.
LLM Quantization
Model quantization is a critical technique in machine learning that reduces the precision of numerical representations. Like converting high-precision floating-point values (FP32, FP16) to lower-precision formats (INT8). Quantization significantly decreases model size, memory usage, and computational demands. This process facilitates the deployment of powerful language models like LLM’s on resource-constrained devices (such as laptop), improving efficiency and accessibility while typically incurring minimal performance degradation. I will write in detail about ML Quantization and techniques in a separate post.
There are different file formats for quantized LLM models. Fig. 7 shows a summarized table of some of these formats. The most famous quantized LLM format is GGUF (GPTQ/GGML Unified Format). It is a file format used for optimized LLM inference, particularly with CPU-based frameworks like llama.cpp, llamafile, and KoboldCpp.
Quantized LLM File Information and Usage
Figure 8 illustrates various quantized Mistral models, ranging from 8-bit to 2-bit, alongside a comparison with the original model. It's evident that as quantization increases, model weight decreases, making the model more suitable for resource-constrained devices. Notably, the 2-bit quantized Mistral 7B model is five times smaller than the original model. However, this size reduction comes with a performance trade-off, which we will discuss in the subsequent section.
Now let’s see what are these different quantization types. Fig. 9 presents a comparison table of different quantization types, detailing their descriptions and performance trade-offs. It highlights the trade-off between accuracy and speed, ranging from 2-bit (fastest but least accurate) to 8-bit (highest accuracy but slower). The table helps in selecting the appropriate quantization level based on optimization needs.
Understanding these trade-offs is crucial, but how do you decide which quantization type to use in practice? To help simplify this decision based on your specific hardware and use case, Fig. 10 provides a summarize guide for selecting the appropriate quantization type based on system specifications. It categorizes use cases from low-RAM (4GB or less) devices to high-performance setups (16GB+ RAM, strong GPUs or CPUs). The recommended quantization levels range from Q2_K for minimal resources to Q8_0 for the highest quality and accuracy.
LLM Serving
Okay, so we've talked about getting these LLMs running inference, which is basically asking them to do their thing. But how do we make that inference available to everyone who wants to use it? That's where LLM serving comes in, and it's a whole different ballgame we need to dive into.
Model serving has emerged as a critical component in the machine learning lifecycle, enabling organizations to transform trained models into valuable business applications. It is the broader infrastructure and system design (Fig. 11) that enables inference at scale and involves deploying the trained model as an API or service (via REST or gRPC APIs) that can handle multiple inference requests from users or applications. Model serving ensures that inference is efficient, scalable, and reliable. Organizations can implement model serving through several architectural patterns, each offering different trade-offs in terms of complexity, resource efficiency, and operational characteristics.
Let's break down how an LLM actually works in a system. Check out Figure 12. You will see the LLM at the heart of it all. To get it to do its thing, we use an inference library – that's the magic behind running the model. You can choose to run it on a CPU or, for a speed boost, a GPU. And to connect everything together, there's an API, acting as the bridge for users and other systems to interact with the LLM.
LLM Serving Frameworks for Different Scenarios
The landscape of LLM serving frameworks has expanded rapidly, with various solutions addressing different aspects of the serving challenge. These frameworks can be categorized based on their primary focus and capabilities, making them suitable for different deployment scenarios.
Lightweight Local LLM Hosting Frameworks
These frameworks are designed to run LLMs efficiently on consumer hardware (like CPU/GPU). They prioritize ease of use and minimal resource consumption, making LLMs accessible to a wider audience without requiring high-end servers. This allows for experimentation and development on personal devices.
Advanced LLM Inference Frameworks
For high-performance LLM hosting on GPUs and multi-node clusters. These frameworks are essential for applications requiring low latency and high throughput, particularly when dealing with large models and complex workloads. They leverage optimized techniques like tensor parallelism, KV Caching, pageAttention and distributed computing to maximize performance.
Local LLM Hosting with Quantization (Optimized for Low RAM & CPU):
These frameworks allow quantization (reducing model size) to run LLMs on low-end machines.
Multi-Model & Distributed LLM Hosting
For multi-GPU and multi-node distributed inference.
LLM UI Frameworks
Building user interfaces for LLMs used to be a real headache. You would have to cobble together a bunch of different tools, which wasn't ideal. Thankfully, we're seeing some great UI frameworks popping up that are specifically designed for LLMs. These frameworks make it easier to build intuitive and interactive interfaces, handling things like complex prompts, streaming responses, and even conversational memory.
Framework Usage Recommendations
In summary, the optimal framework for hosting your local LLM depends heavily on your specific needs and available resources (see Fig. 18). Whether you require a simple, local setup, advanced GPU inference, or a distributed, multi-GPU solution, the landscape offers diverse tools like Ollama, vLLM, and DeepSpeed. By carefully considering your use case and hardware, you can effectively deploy and leverage the power of large language models within your own environment.
Practical Usage
Now let’s see some methods to load these LLM models and Perform Inference-
Hugging face Transformer Library
The Hugging Face Transformers library is a powerful tool that makes working with large language models (LLMs) much easier. It's like a toolbox filled with pre-built models and tools, letting you quickly use and adapt cutting-edge AI. Whether you're interested in text generation, translation, or understanding language, Transformers simplifies the process. Lets see how it works
from transformers import AutoModelForCausalLM, AutoTokenizer
import torch
# path to your locally downloaded Mistral 7B model
local_model_path = "/Users/piyush/Desktop/Codes/model/Mistral-7B-Instruct-v0.3"
# load the tokensizer from the local directory
tokenizer = AutoTokenizer.from_pretrained(local_model_path)
# check hardware cpu/gpu or mps for apple
device = "mps" if torch.backends.mps.is_available() else "cpu"
# load model
model = AutoModelForCausalLM.from_pretrained(
local_model_path,
torch_dtype=torch.float16, # Use float16 for lower memory usage
device_map={"": device} # Load on Apple Metal GPU if available
)
# Function to generate text
def chat(prompt):
inputs = tokenizer(prompt, return_tensors="pt").to(device)
with torch.no_grad():
outputs = model.generate(**inputs, max_new_tokens=100)
return tokenizer.decode(outputs[0], skip_special_tokens=True)
# Test the model
prompt = "Which part of Indian cosntitution is borrowed from the Ireland?"
response = chat(prompt)
print(response)
The output above displays the response generated by the Mistral 7B 16-bit model, using the Hugging Face Transformers library. You'll notice a sharp increase in memory usage (video) when the model is loaded. As mentioned before, it's important to choose models that match your hardware and intended use.
llama.cpp
Llama.cpp is a lightweight and efficient library designed to run LLMs on CPUs, even with limited resources. It excels at running models in GGUF format, making it ideal for devices with low RAM. This library allows users to experience the power of LLMs without requiring dedicated GPUs, broadening accessibility to these powerful AI tools. Let’s see how it works-
from llama_cpp import Llama
# laod the mistrl gguf model
llm = Llama(
model_path="/Users/piyush/Desktop/Codes/model/Mistral-7B-Instruct-v0.2-GGUF/mistral-7b-instruct-v0.2.Q8_0.gguf"
)
# define user and system prompts
messages = [
{"role": "system", "content": "You are an expert assistant"},
{"role": "user", "content": "Explain the concept of quantum computing in simple terms."}
]
# Generate response
response = llm.create_chat_completion(messages=messages)
# Extract and print the assistant's reply
generated_text = response["choices"][0]["message"]["content"].strip()
print("Assistant:", generated_text)
vllm and Ollama
If you're working on a Linux system, vLLM is an excellent option for efficient LLM inference on GPU. However, macOS support for vLLM is currently limited. As of today, achieving optimal performance on macOS typically requires building vLLM from source, which can be a more complex process.
Ollama is best to use for general audience with a one liner commad
ollama run mistral <or any other model>
Conclusion
I hope this overview has given you a clearer picture of LLMs, from their inner workings to practical deployment. We've covered a lot of ground, but this is just the beginning! In the next series of posts, we'll dive into building a real-world LLMOps framework. We'll walk through the process of creating an API and a chat UI, so you can interact with your LLM. Thanks for reading !!.
References
Sculley, David, Gary Holt, Daniel Golovin, Eugene Davydov, Todd Phillips, Dietmar Ebner, Vinay Chaudhary, Michael Young, Jean-Francois Crespo, and Dan Dennison. "Hidden Technical Debt in Machine Learning Systems."1 Advances in Neural Information Processing Systems 28 (2015).2
"MLOps for Better Machine Learning Deployment." Codilime Blog. Accessed. https://codilime.com/blog/mlops-for-better-machine-learning-deployment.
Remthix, S. D. "Discovering MLOps." Medium. Accessed. https://sdremthix.medium.com/discovering-mlops-a49ef3139696.
"What Is LLMOps?" Pluralsight Resources Blog. Accessed. https://www.pluralsight.com/resources/blog/ai-and-data/what-is-llmops.
"LLMOps." Databricks Glossary. Accessed. https://www.databricks.com/glossary/llmops.
"LLMOps vs. MLOps: Understanding the Differences." Iguazio Blog. Accessed. https://www.iguazio.com/blog/llmops-vs-mlops-understanding-the-differences/.
"LLMOps." lakeFS Blog. Accessed. https://lakefs.io/blog/llmops/.
https://www.datacamp.com/tutorial/quantization-for-large-language-models
AI Engineering. O'Reilly. Accessed. https://www.oreilly.com/library/view/ai-engineering/9781098166298/.
"Transformers Documentation." Hugging Face. Accessed. https://huggingface.co/docs/transformers/en/index.
"vLLM CPU Installation on Apple Silicon." vLLM Documentation. Accessed. https://docs.vllm.ai/en/latest/getting_started/installation/cpu.html?device=apple.
"Ollama." Accessed. https://ollama.com/
."koboldcpp." GitHub. Accessed. https://github.com/LostRuins/koboldcpp.
"LlamaIndex." Accessed. https://www.llamaindex.ai/
"LangChain." Accessed. https://www.langchain.com/
"Haystack." Deepset AI. Accessed. https://haystack.deepset.ai/
"LM Studio." Accessed. https://lmstudio.ai/
"Streamlit." Accessed. https://streamlit.io/
"llama.cpp." GitHub. Accessed. https://github.com/ggml-org/llama.cpp.
"exllama." GitHub. Accessed. https://github.com/turboderp/exllama.
"Open WebUI." Accessed. https://openwebui.com/
"OpenLLM." GitHub. Accessed. https://github.com/bentoml/OpenLLM.